간단한 예제 프로그램 실행
1) hdfs 확인
2) Jar 파일 생성
WordCountMapper.class
WordCountReducer.class
WordCount.class
3) Jar 파일 실행
4) 결과 확인
간단한 예제 프로그램 실행
하둡을 다운로드 하면, tar 폴더안에 함께 포함되어 있는 wordcount jar 파일이 존재한다.
해당 파일을 사용하여 테스트를 진행해 보자.
1) hdfs 확인
-
hdfs 에 기본 경로 폴더 생성
log@ubuntu:~/hadoop/hadoop-3.1.3$ bin/hdfs dfs -mkdir /user log@ubuntu:~/hadoop/hadoop-3.1.3$ bin/hdfs dfs -mkdir /user/log log@ubuntu:~/hadoop/hadoop-3.1.3$ bin/hdfs dfs -mkdir /user/log/test log@ubuntu:~/hadoop/hadoop-3.1.3$ bin/hdfs dfs -ls
-
mapreduce 테스트를 위해 harrypoter.txt파일을 로컬에 생성한다. txt 파일 안에 내용은 구글링해서 나오는 위키백과의 내용을 복사 붙여넣기 했다.
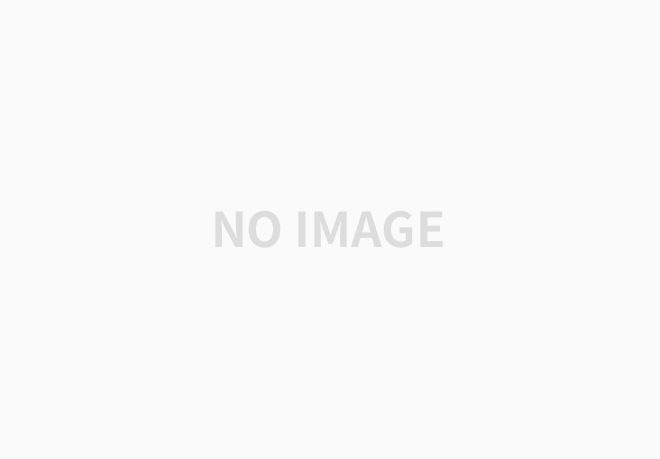
log4@ubuntu:~/hadoop/hadoop-3.1.3/sample$ sudo nano harrypoter.txt
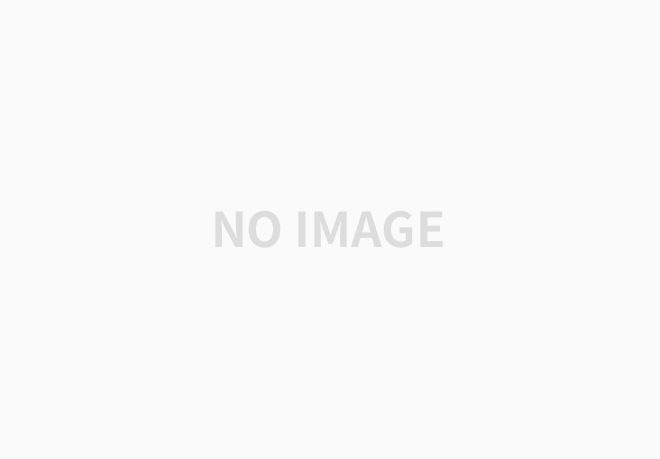
- harrypoter.txt 파일을 hdfs에 넣고, 잘 들어 갔는지 확인한다.
log4@ubuntu:~/hadoop/hadoop-3.1.3/sample$ hdfs dfs -put harrypoter.txt
log4@ubuntu:~/hadoop/hadoop-3.1.3/sample$ hdfs dfs -ls
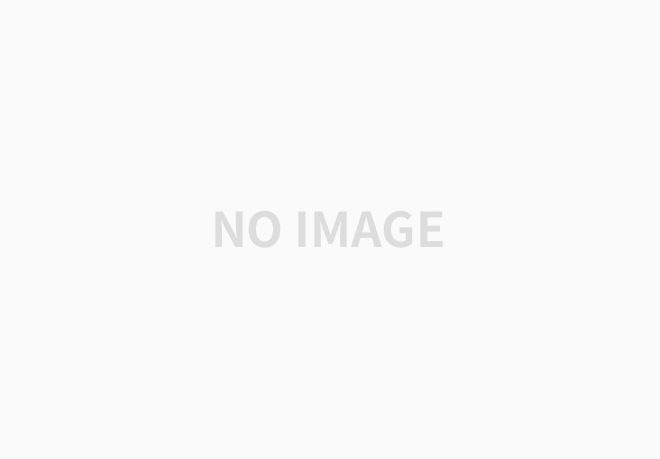
2) Jar 파일 생성
hadoop에서는 sample로 map-reduce jar 파일을 제공하지만, 이 실습에서는 간단한 맵리듀스를 클래스를 생성하여 만든 jar 파일로 진행한다.
간단한 맵리듀는 아래의 3가지 클래스로 구성된다.
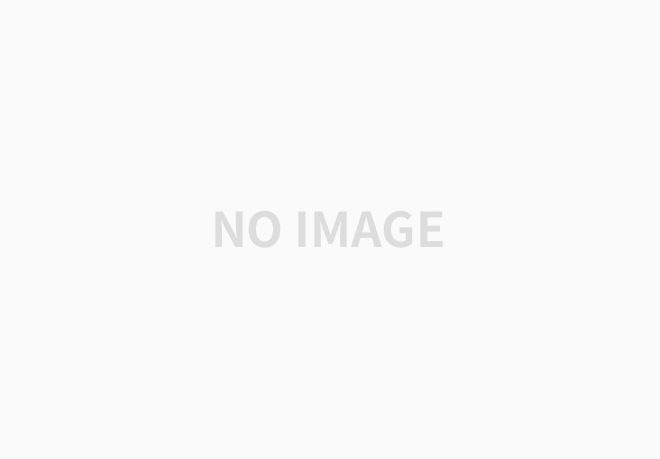
WordCountMapper.class
package hadoop;
import org.apache.hadoop.io.IntWritable;
import org.apache.hadoop.io.LongWritable;
import org.apache.hadoop.io.Text;
import org.apache.hadoop.mapreduce.Mapper;
import java.io.IOException;
import java.util.StringTokenizer;
// input : <들어온 순서, 라인 단위 텍스트>
// output : <끊은 단어, 1>
public class WordCountMapper extends Mapper<LongWritable, Text, Text, IntWritable> {
// 결과 값으로 보낼 값으로 1로 고정
private final static IntWritable one = new IntWritable(1);
// 하둡에서는 String 자료형을 하둡전용 자료형인 Text를 사용한다.
private Text word = new Text();
public void map(LongWritable key, Text value, Context context) throws IOException, InterruptedException {
//공백 단위로 끊어내어서, 그 값을 output key로 정하고 그 밸류는 1로 고정한다.
StringTokenizer itr = new StringTokenizer(value.toString());
while (itr.hasMoreTokens()){
// word에 단어를 넣는다.
word.set(itr.nextToken());
// 리듀스로 보내는 작업
context.write(word, one);
}
}
}
WordCountReducer.class
package hadoop;
import org.apache.hadoop.io.IntWritable;
import org.apache.hadoop.io.Text;
import org.apache.hadoop.mapreduce.Reducer;
import java.io.IOException;
// 맵의 output 데이터 형식과 리듀스의 input 데이터 형식은 같아야한다.
public class WordCountReducer extends Reducer<Text, IntWritable, Text, IntWritable> {
private IntWritable result = new IntWritable();
protected void reduce(Text key, Iterable<IntWritable> values,
Reducer<Text, IntWritable, Text, IntWritable>.Context context) throws IOException, InterruptedException {
int sum = 0;
// 동일한 단어의 합을 구한다.
for (IntWritable val : values) {
sum += val.get();
}
result.set(sum);
context.write(key, result);
}
}
WordCount.class
package hadoop;
import org.apache.hadoop.conf.Configuration;
import org.apache.hadoop.fs.Path;
import org.apache.hadoop.io.IntWritable;
import org.apache.hadoop.io.Text;
import org.apache.hadoop.mapreduce.Job;
import org.apache.hadoop.mapreduce.lib.input.FileInputFormat;
import org.apache.hadoop.mapreduce.lib.input.TextInputFormat;
import org.apache.hadoop.mapreduce.lib.output.FileOutputFormat;
import org.apache.hadoop.mapreduce.lib.output.TextOutputFormat;
import java.io.IOException;
public class WordCount {
public static void main(String[] args) throws IOException, ClassNotFoundException, InterruptedException {
Configuration conf = new Configuration();
if(args.length != 2){
System.out.println("Usage : WordCount <input> <output>");
System.exit(2);
;
}
Job job = new Job(conf, "WordCount");
// jar 파일 실행 클래스 설정
job.setJarByClass(WordCount.class);
// 매퍼 클래스 설정
job.setMapperClass(WordCountMapper.class);
// 리듀스 클래스 설정
job.setReducerClass(WordCountReducer.class);
// 입출력 데이터 포맷 설정
job.setInputFormatClass(TextInputFormat.class);
job.setOutputFormatClass(TextOutputFormat.class);
//매퍼와 리듀스 클래스의 출력 데이터의 키와 값 타입을 설정
job.setOutputKeyClass(Text.class);
job.setOutputValueClass(IntWritable.class);
// 입출력 데이터를 어떤 경로로 전달 받은 것인지 설정.
FileInputFormat.addInputPath(job, new Path(args[0]));
FileOutputFormat.setOutputPath(job, new Path(args[1]));
// 잡 객체의 waitForCompletion메서드를 호출해 잡을 실행
job.waitForCompletion(true);
}
}
만약 Intellij 라면, Build > Build Artifacts...
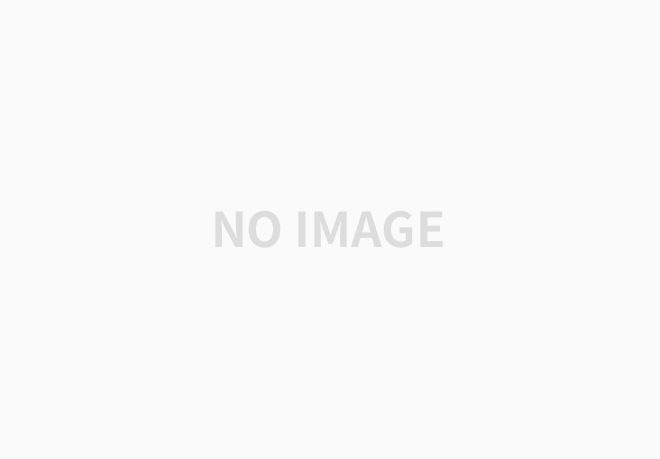
jar 파일 생성관련해서 추가적으로 정리하겠습니다.
3) Jar 파일 실행
생성한 jar 파일을 hadoop 명령어를 통해 실행한다.
명령어 형식
hadoop jar {jar file} {input file} {output file}
log4@ubuntu:~/hadoop/hadoop-3.1.3$ hadoop jar WordCount.jar harrypoter.txt output3
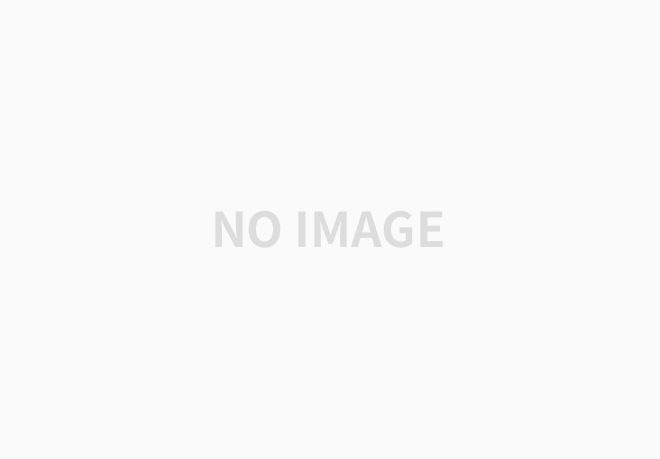
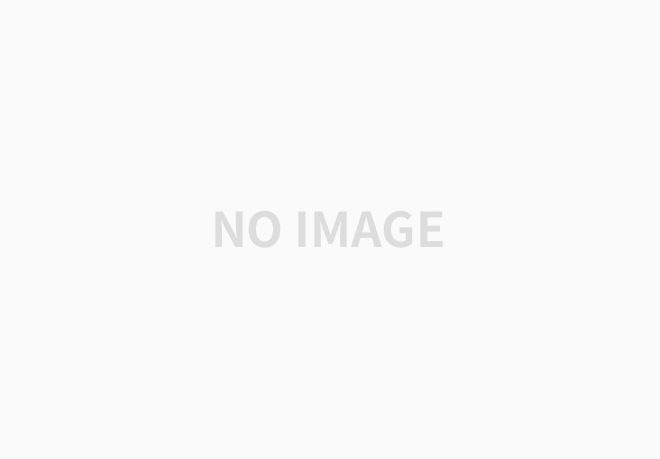
4) 결과 확인
hdfs에 output3 폴더가 잘 생성되었는지 확인해 보자.
log4@ubuntu:~/hadoop/hadoop-3.1.3$ hdfs dfs -ls
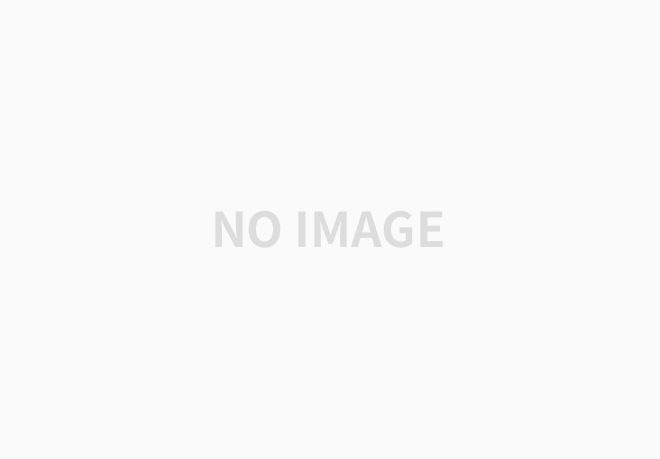
output3폴더 안에 들어가서 맵 리듀스 결과도 확인해보자.
log4@ubuntu:~/hadoop/hadoop-3.1.3$ hdfs dfs -ls output3
log4@ubuntu:~/hadoop/hadoop-3.1.3$ hdfs dfs -cat output3/part-r-00000
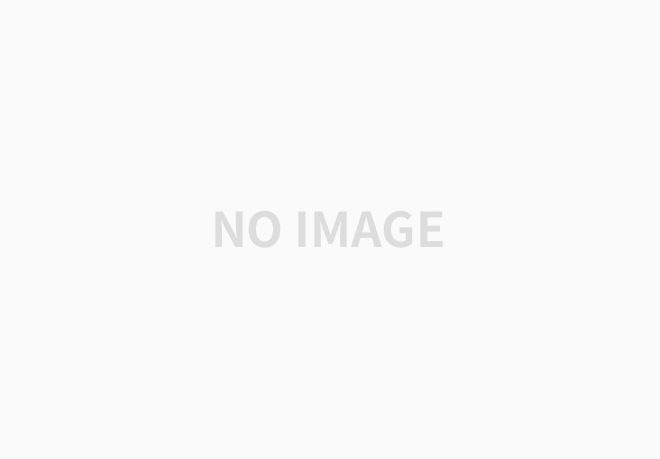
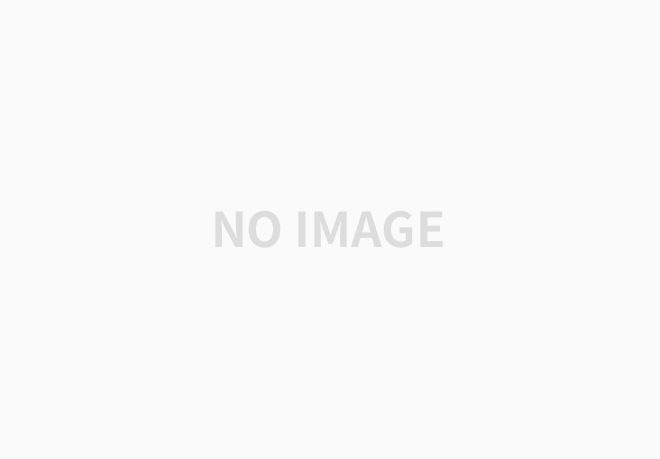
cat 명령어를 사용하여 내용을 확인해보면, 공백 기준으로 구분한 단어들의 합계를 출력 된것을 확인할 수 있다.
'Programming > Hadoop' 카테고리의 다른 글
[Hadoop] Window NameNode & DataNode 실행 오류 (0) | 2020.09.23 |
---|---|
[Hadoop] Ubuntu 18.04 + Hadoop 3.1.3 Setting On Linux (0) | 2020.09.19 |
[Hadoop] hdfs -ls 오류 (0) | 2020.09.19 |